Autonomous AI systems that make decisions and manage processes independently? What sounds like science fiction is quickly becoming the next major innovation field for banks. In this article, Bala Nagaraj explains why AI agents are particularly relevant right now, which technological foundations are already in place, and how financial institutions can benefit. A look at the opportunities, challenges, and the next level of digitalization in the financial sector.
Why AI agents are so relevant right now
They are considered the “Next Big Thing” in AI development: AI agents. What may still sound futuristic is already becoming reality – and offers tremendous opportunities for banks. This article explains what defines AI agents, their stages of development, and how banks can already benefit technologically, strategically, and operationally.
AI agents are autonomous software entities that perceive their environment, make decisions, and take actions to achieve specific goals. The key difference compared to earlier AI systems: they act largely independently and require minimal human approval for the final step. This sets them apart from co-pilot systems or the human-in-the-loop approach, where AI merely provides suggestions that humans must review or approve.
Thanks to the rise of powerful large language models (LLMs) and standardized interfaces (APIs), the technological conditions are now in place to not just conceptualize but actually implement AI agents. Agents can access existing tools, interpret environmental information, and act accordingly. This not only opens new efficiency potentials but also has the power to transform entire processes, roles, and business models in finance.
What defines AI agents
To truly understand the potential of this technology, it is worth looking under the hood. A typical AI agent consists of:
- a toolset for interacting with the external world, such as databases, web interfaces, or robot control systems,
- a memory to learn from past decisions,
- an environment interface to perceive context (data streams, sensors, or other inputs that help the agent understand and react to its environment),
- and a brain – usually a powerful LLM – that makes decisions.
Maturity levels: From rule-based to highly intelligent
AI agents evolve through several stages – from simple rule-based tools to learning, collaborative systems. We are currently operating mostly within the mid-level stages (Level 2–3). Here’s an overview of the six levels showcasing the tremendous development potential:
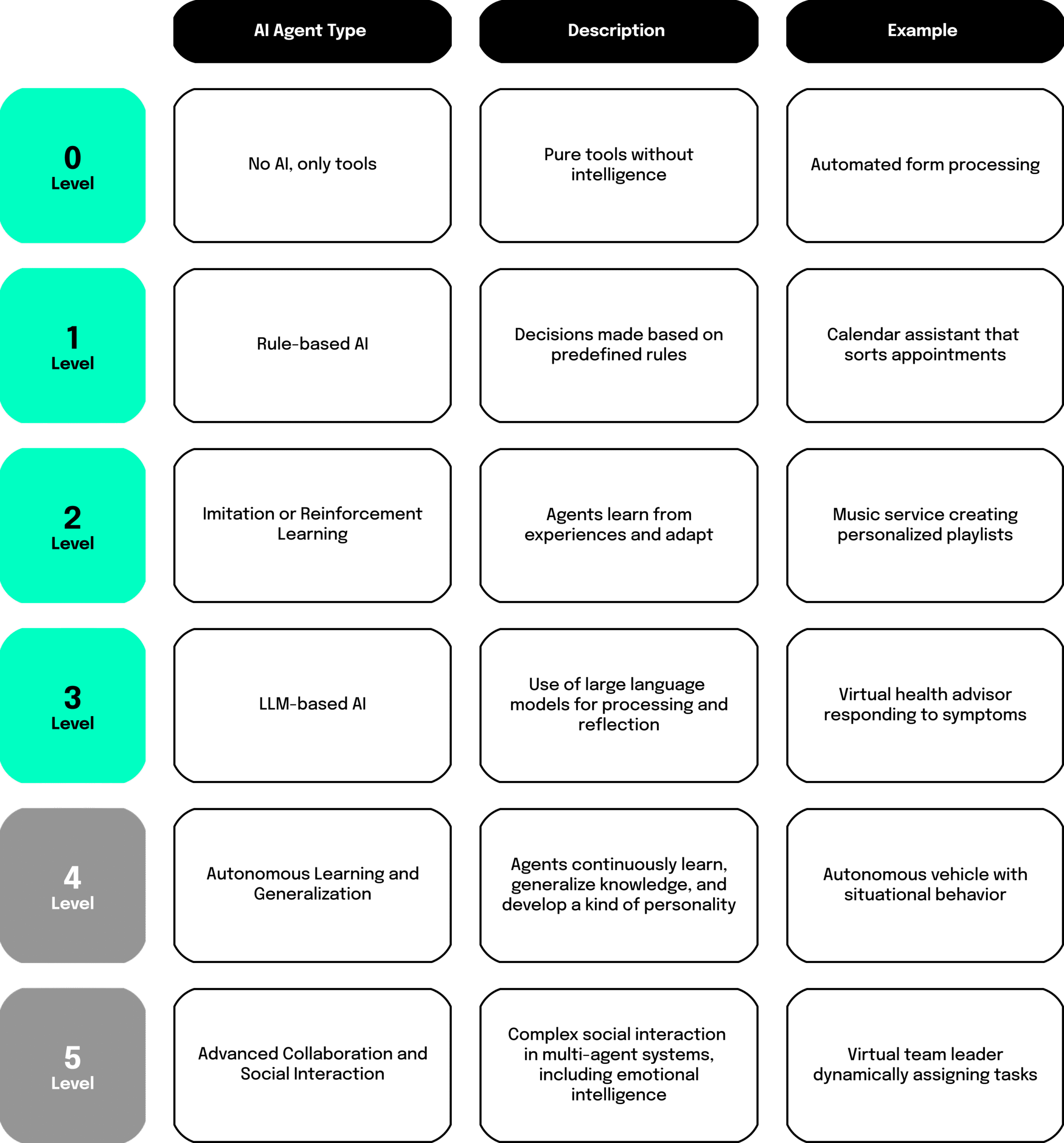
How the AI agent ecosystem is forming
AI agents are not a finished product but a technology – and thus not a clearly defined market. Still, the emerging ecosystem and investments are worth a look: in 2024, over $100 billion were invested globally into AI startups – many with a focus on AI agents.
The ecosystem currently forms across three layers:
- Foundation Layer: Includes technological infrastructure like runtime environments, APIs, and large language models such as ChatGPT (OpenAI), Claude (Anthropic), and Gemini (Google).
- Agent Platforms: Frameworks and toolkits for building, combining, and orchestrating AI agents. Examples: LangChain (development & orchestration), AgentOps (monitoring & management), CrewAI (multi-agent coordination).
- Application Layer: Ready-to-use AI agents for specific use cases, often as SaaS solutions. Examples: PolyAI (voice bots), Numbers Station (data analysis), Finster AI (investments), Lovable (app development).
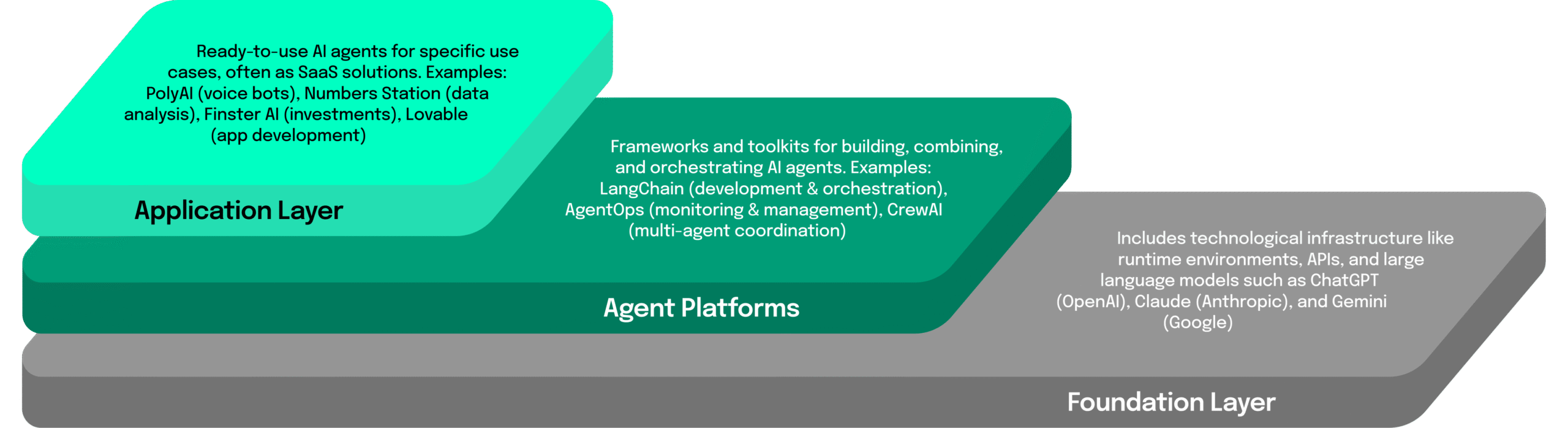
Where AI agents create true value in banking
AI agents offer banks a wide range of applications – internally for improving processes and products, and externally for redefining the customer experience.
A compelling example is the loan application process – a data-intensive procedure with many steps. Here, AI agents can add value across the entire chain:

The big leap is not just automating single steps but orchestrating them. When specialized agents collaborate meaningfully, they create an adaptive, scalable end-to-end process – going beyond individual products.
For customers, the experience may no longer feel personal but will be highly personalized – often even more so than with human advisors.
Why AI agents need clear rules and control
Despite all their potential, implementing AI agents brings technological, regulatory, and ethical challenges. Especially because these systems act autonomously, new control mechanisms and realistic expectations are crucial.
- Data Quality: The quality of decisions depends directly on the quality of data. Outdated or biased data leads to errors.
- Bias and Fairness: Like all LLM-based systems, AI agents carry the risk of bias – through training data or implicit assumptions. In finance, this can result in discriminatory outcomes. Banks must be aware of their own biases to prevent passing them on to AI.
- Mistakes: AI agents can make mistakes, especially in unfamiliar situations. Feedback loops and clear intervention processes are necessary.
- Regulatory Compliance: Automated decisions are subject to strict rules. Data protection, consumer protection, and supervisory obligations must be respected – even as regulations themselves are still evolving.
- Control Mechanisms: Since AI agents make decisions independently, overarching structures are needed – e.g., supervisor agents or human-in-the-loop instances for critical applications.
Banks can only unlock the full potential of AI agents if they address these challenges seriously while exploring new paths. Progress comes not from theory but through practical action, testing, reflecting, and learning.
What banks can do now – and what's coming next
Even though developments around AI agents are still in their early stages, banks can already take action. Three strategic areas stand out:
- Identify High-Impact Use Cases: Rather than scaling up immediately, banks should focus on processes with the highest potential, such as lending, compliance, or customer engagement.
- Prepare Agent Infrastructure: An early, modular, and secure architecture lays the groundwork for future scaling. A strong data foundation, transparent logic, and a quality assurance framework are critical.
- Start With Partners and Pilots: Collaborating with open-source initiatives, startups, or innovation teams allows for practical, low-risk pilots with high learning potential.
Beyond these first projects, several perspectives are emerging:
- Hyperpersonalization: AI agents will dynamically and individually tailor products, communication, and decisions – far beyond traditional marketing.
- Agents as the Customer Interface: Customer interaction with banks could shift to agent-to-agent communication – on both sides.
- Disruption of Entire Processes: Agents could not only advise but also execute and continuously improve processes, fundamentally changing business models.
Today I was sent the following cool demo:
Two AI agents on a phone call realize they’re both AI and switch to a superior audio signal ggwave
pic.twitter.com/TeewgxLEsP— Georgi Gerganov (@ggerganov)
February 24, 2025
Preparing for a different tomorrow
The development of AI agents is rapid – both technologically and practically. Yet we are still at the beginning. Many questions remain open, and much will only become clear through practical experience – which will inevitably raise new questions.
That’s why now is the right time to get started. Not with the expectation of perfection, but with the goal of gaining experience, understanding risks, and unlocking opportunities responsibly.
Because one thing is certain: The financial world of tomorrow will be different from today – and it will be shaped by those willing to take new paths today.
To succeed, banks need a realistic attitude: a balance between vision and feasibility. Neither blind belief in progress nor rigidly clinging to the status quo will secure the future.
The pace of change is accelerating – and demands above all one thing: adaptability. From every bank. Including yours.
More about artificial intelligence here!
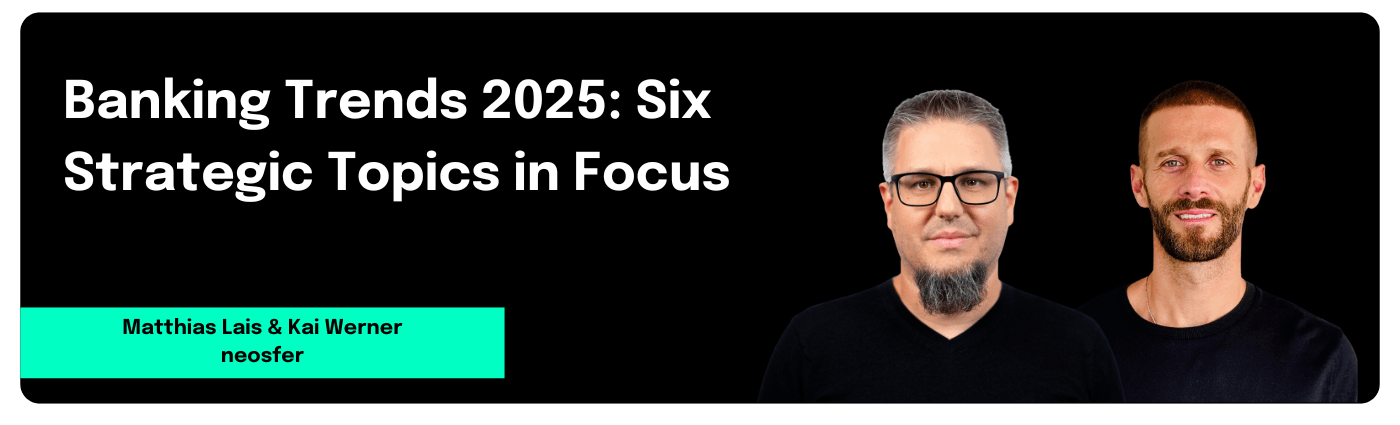
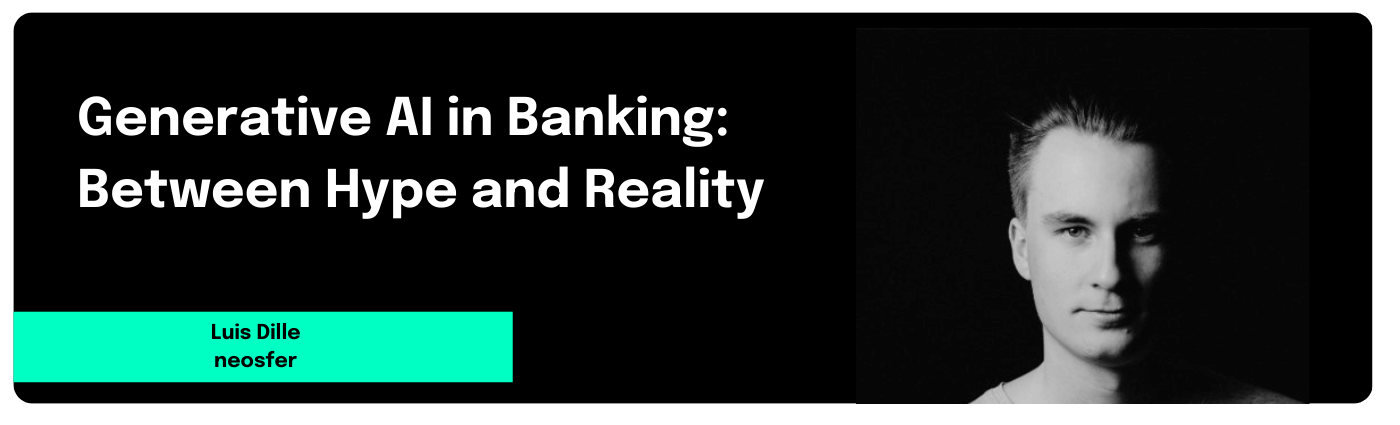
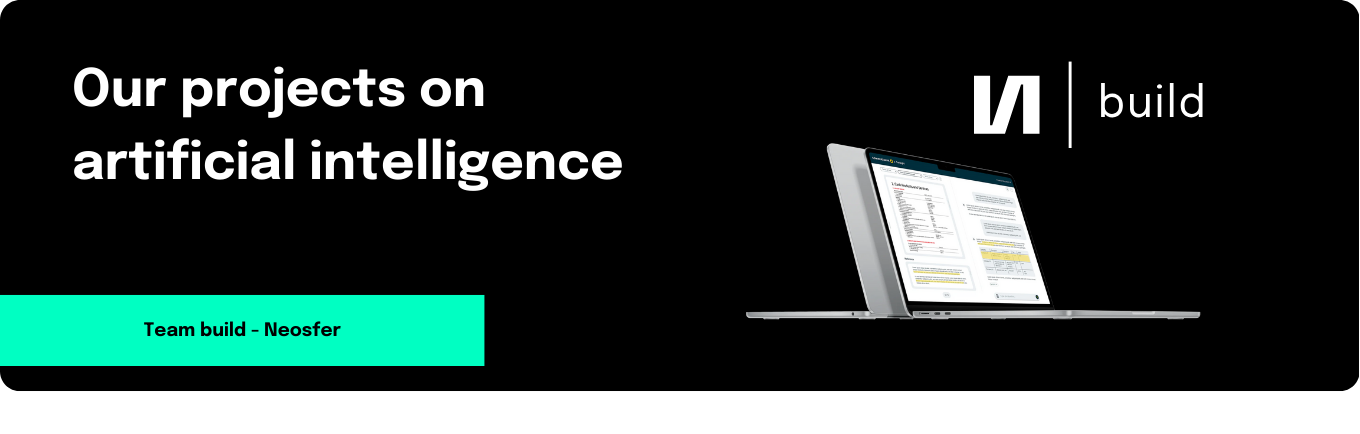