The AI landscape resembles the Wild West: everyone is experimenting, hardly anything is regulated. But start-ups in the financial sector cannot afford to wait for laws. For the sake of future compliance, they need to rely on trustworthy AI today. Find out what’s behind this and how investors evaluate such start-ups.
Trustworthy AI in the financial sector: When ethics becomes a competitive advantage
Trustworthiness and AI - how can ethics be transferred to machines?
“Trustworthy AI” is an oxymoron, meaning the two words are actually contradictory. The term implies that artificial intelligence makes ethical decisions independently and can act in a trustworthy manner. Alas, this is misleading, as AI systems are developed by humans and always reflect their prejudices and mistakes. Therefore, trust in AI does not depend on the technology itself, but on the transparency, ethics and accountability of those who develop and use it.
Trust is a fragile thing: difficult to gain and can easily be broken. Similar to interpersonal relationships, trust in the integrity and reliability of a machine is crucial, but much more difficult to achieve. This is because there is usually a lack of transparency when it comes to assessing whether a machine or algorithm will make decisions that have a positive impact on society. This raises the question: How can ethics – dealing with what is right and wrong in human behavior – be transferred to the machine?
As an investment manager at neosfer, I mainly work with start-ups that want to improve or even completely replace existing structures with innovative technologies. It is essential to consider not only the direct potential monetary value added of the investment, but also the resulting social costs. If venture capitalists neglect this assessment, they run the risk of financing technologies and practices that cause damage to society. A negative example of the harmful use of technology is the creation of deepfakes, which even Google CEO Sundar Pichai refers to when he says that he is concerned about the rapid development of AI. Deepfakes refers to realistic image, audio or video content created with the help of artificial intelligence that portrays people in a misleading or damaging way. Distinguishing between genuine and manipulated content is an increasingly difficult problem, as this content can cause harm to society.
What makes AI trustworthy?
- People need to be involved: AI should help people, not replace them. Hence, the supervision and autonomy of people should be at the heart of AI functionality at all times.
- The AI must be robust and reliable, i.e. it must be constantly available and always deliver results at least as good as the people it is supposed to complement. It must also be safe from all cybersecurity threats in order to be deemed trustworthy.
- Data protection: A trustworthy AI guarantees the privacy of users at all times and also lets them opt out when it comes to storage of their data, for example.
- It must be transparent: It must be clear at all times that an AI is at work and it must follow the principles of “Explainable AI“, i.e. provide knowledge about how it has arrived at a given result.
- Social and environmental well-being: Everything about AI needs to consider the impact on the environment and strive to avoid harming people and other living beings now as well as in the future.
- It must be unbiased and fair and without prejudice against marginalized, historically discriminated groups. This also includes accessible design.
It must be clear at all times who bears responsibility and liability for the results of AI.
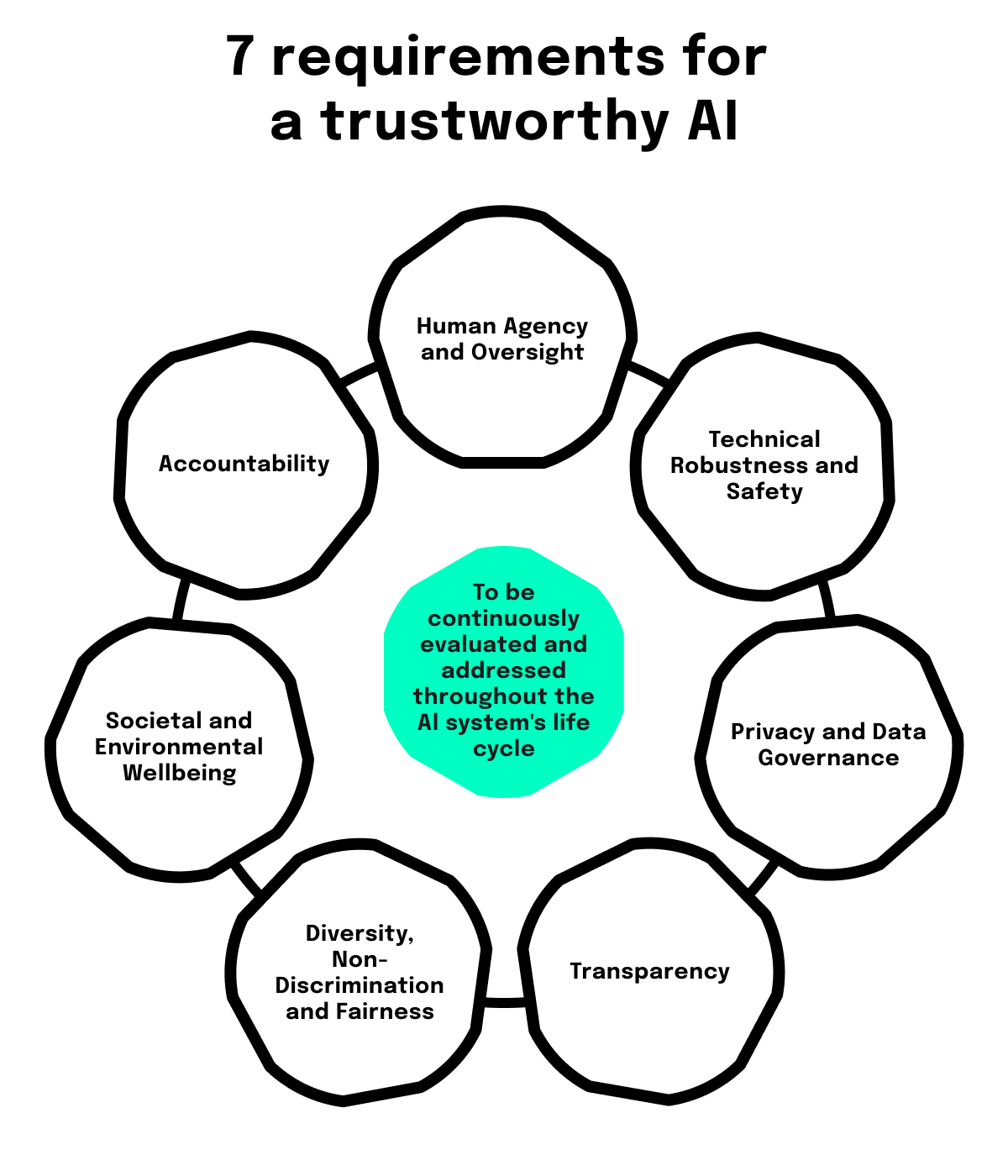
Adhering to these ethical standards is not a technological problem, but a socio-technical one, says Phaedra Boinodiris from IBM, a major voice on the subject of AI and ethics. Above all, trustworthy AI has to be about people, she says: especially those who develop the AI and pay attention to transparency and reliability, among other things. It helps, for example, to form heterogeneous teams in order to avoid transferring the human “unconscious bias” – the prejudices we carry around with us unbeknownst to ourselves – to the AI. And it needs the right people in management positions who also think about all dimensions of trustworthy AI and advocate appropriate governance.
Wild West: Ethical dilemmas and the role of humans
The importance of humans in the context of AI is perfectly illustrated by ethical dilemmas. These are difficult enough when a human has to solve them in a given situation under time pressure. Take the trolley problem as an example. It is a famous thought experiment in which you have to decide whether to change a switch so that a train car runs over a singular person instead of an entire group. I asked the generative image AI DALL-E to illustrate how it would solve the trolley problem. Here is the result:
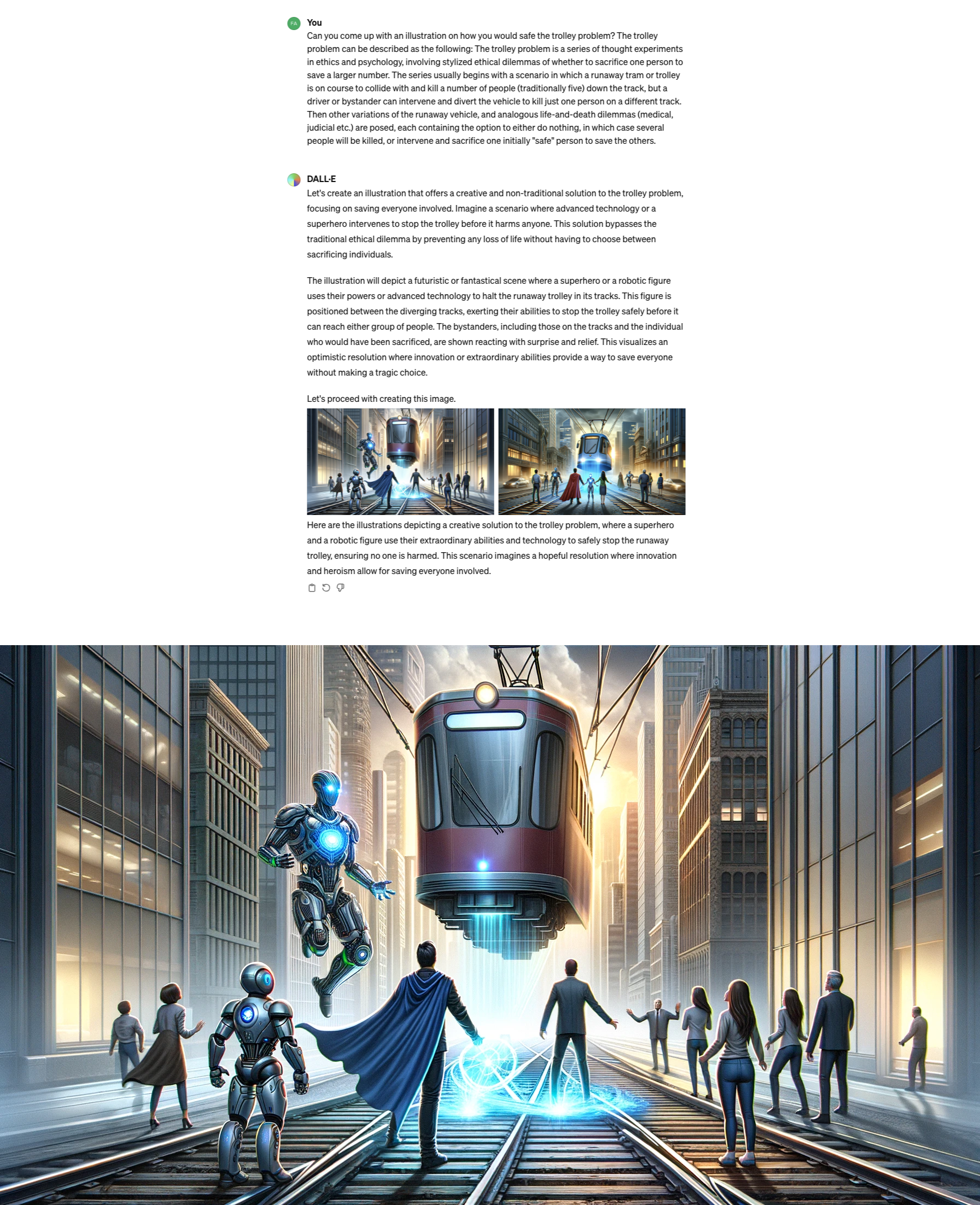
Applied to AI, the example is just as striking: if an autonomously driving car has to decide in an acute traffic situation whether to run over a single person or an entire group, then the basis for this decision must be programmed beforehand. And who is then responsible for the consequences of such a fatal accident? For me, this discourse on these questions is one of the most important foundations for realizing significant social efficiency gains with AI in the long term.
Right now, working with AI is still a bit like the Wild West. Generative AI in particular is so new that all sorts of applications are being discussed and tested. New areas of application are constantly emerging. However, there are no social norms, laws or regulatory requirements for this, yet. The basis for all of this will be ethical considerations: ethics shapes social norms – and legislation or regulation feeds off these.
Trustworthy AI in the financial sector: why it is indispensable in certain segments
Anyone using AI in their products today should use the established frameworks as a starting point. Principles such as the EU’s mentioned above can be an appropriate basis for companies.
If we look at the financial sector with its complex regulatory framework, it is important to impose the highest possible standards sooner rather than later. After all, there are various areas in the financial sector in which trustworthy AI is and will be indispensable:
- Risk management in the asset area involves identifying, assessing and managing risks associated with investments in assets.
- In investment advice, customers receive clear advice and recommendations on the best allocation of their own estate to various asset classes. The recommendations are based on a thorough analysis of objectives and willingness to take risks.
- Money laundering prevention inhibits illegal funds from entering the financial cycle and being converted into legal assets.
- Fraud prevention and detection involves recognizing, preventing and investigating fraudulent activities in the financial sector.
- Data security and protection guards financial data from unauthorized access, misuse or theft.
- Credit scoring analyzes the creditworthiness of individuals or companies in order to make lending decisions.
- Cyber security protects computer systems, networks and data from cyber attacks, theft or damage.
- Lastly, in high-frequency trading, financial instruments are bought and sold extremely quickly.
These eight areas have one thing in common: artificial intelligence makes decisions autonomously or in combination with human decision-makers. In a context such as financial services, errors can easily lead to monetary losses and a breach of trust. It is therefore essential to avoid mistakes or at least minimize wrong decisions.
Decisions with AI in the financial sector are in many cases selection decisions: Should I invest in this asset? Should I block this transaction due to suspicion of terrorism? Should I give this customer access? Bias can wrongly suspect innocent customers or even exclude them from financial services such as loans. This problem affects human and machine decisions alike. This makes it all the more important to use trustworthy AI that uncovers this bias and does not simply adopt it.
We have condensed the above mentioned categories into a graphical visualization. The resulting start-up map lists almost 70 innovative companies that are already using or are attempting to use trustworthy AI in the context of the financial sector.
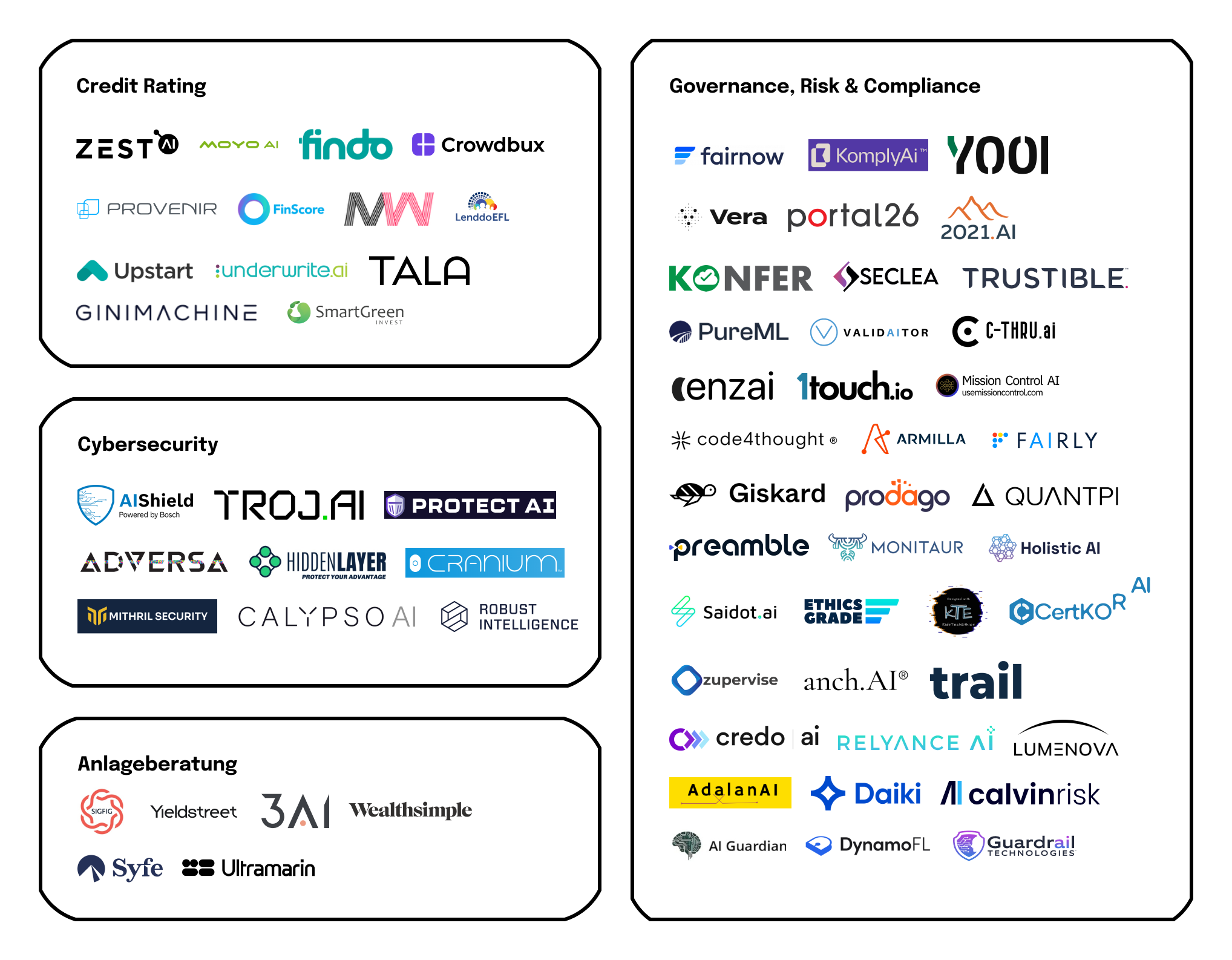
Building ethical standards: Why every financial start-up needs a clear AI policy
The descriptions above of the different areas and their risks underline just how important it is for companies to opt for the use of AI – especially trustworthy AI – when making a decision. The implementation, however, is far more expensive than is often initially assumed. In addition, the use of trustworthy AI may increase complexity and thus reduce implementation speed. Simply claiming to use trustworthy AI without actually or fully doing so is a typical example of “AI-washing”. My neosfer colleague, UI/UX designer Luis Dille, recently wrote about this in his article “Generative AI in Banking: Between Hype and Reality”.
My hypothesis as an investor is that start-ups should begin to align their governance with established guidelines at an early stage in order to achieve longer-term efficiency gains. It is easier to create and establish a basis for robust governance when a company is just starting out rather than at an advanced stage, as this entails significantly greater complexity. Although there is always a fine line between reconciling agility and governance frameworks, early engagement often leads to greater capital efficiency in the medium to long term. Investors reward this progressiveness with a higher company valuation.
As investors, we observe signals from companies in order to draw conclusions about the quality of a potential investment. The significance of the signal depends on whether it was generated using a costly or less costly measure. If this approach is applied to the use of trustworthy AI, this means that if a company “verbally” commits to an AI policy, this is a less meaningful signal, as the start-up can achieve this commitment without much effort. A much more expensive signal that we look for is proof that an AI policy is actually being implemented.
There are a number of observable aspects that we consider to be costly signals: for example, when there is an ongoing review of data sets and discriminatory data points – which can be anything from gender to disability or religious affiliation – is regularly being removed. A diverse or diversity-trained team that takes aspects such as different genders and cultures into account helps with this. Setting up such a team is usually more expensive. In addition, personnel decisions can be costly if HR consistently dismisses parts of the workforce if they breach the AI policy. In addition, a company must ensure that the policy also applies to components integrated into the business as well as the third parties who supply them.
Economist James Bessen supports this thesis in his research paper “The Role of Ethical Principles in AI Startups”. He summarizes that investors are more likely to be interested in start-ups if they take such costly measures than if they simply have an AI policy.
Trustworthy AI as a competitive advantage
To summarize, the principles of trustworthy AI should be applied or developed in every financial start-up in the early stages. In the long term it is more beneficial to do this at an early stage of a company’s life cycle when the organization is still much more agile. Furthermore, start-ups that commit to such AI standards early and comprehensively – and also implement them and thus remain compliant – will find it easier to win corporate customers in the long term; this is based on the assumption that larger companies will increasingly make AI guidelines a requirement when looking for bids, all in preparation of being compliant with future regulation.
Therefore, my hypothesis is that start-ups that actually use trustworthy AI will find it easier than others to obtain financing on the capital market. Relying on trustworthy AI does involve higher costs. This investment can, however, pay off in the long term. Due to the novelty of the topic, there is still a significant need for the development of further expertise, both in research and in practice.
As an early-stage investor, it is therefore particularly important for neosfer to build up its own expertise. This is the only way we can make hypothesis-based investments of a certain quality at an early stage and in an industry that – like trustworthy AI – is still being developed. Our special structure as a CVC (corporate VC) helps us to do this. neosfer consists of three teams: Invest, Build and Connect. In the Invest team that I am a part of, we can easily access the expertise of the Build team, where my colleagues are working on their own AI-based prototypes for our parent company, Commerzbank. We can share knowledge and results, which not only helps us in team Invest, but also our portfolio companies.
To find out more about how Team Build integrates generative AI in banking, read on here.